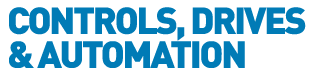
![]() |
Charlotte Stonestreet
Managing Editor |
Home> | IIot & Smart Technology | >Big Data | >Improving predictive maintenance with artificial intelligence |
Home> | AUTOMATION | >Condition Monitoring | >Improving predictive maintenance with artificial intelligence |
Home> | IIot & Smart Technology | >Industry 4.0 | >Improving predictive maintenance with artificial intelligence |
Improving predictive maintenance with artificial intelligence
30 June 2022
Steve Sands observes the changes in this technology sector and the impact on customers

TODAY'S MANUFACTURERS of automation machinery need to differentiate themselves by creating added value for their customers. Digitalisation solutions provide the most significant opportunity for our customers to improve their machinery's productivity performance and differentiate themselves from their competitors. As a solutions-led company with more than 300,000 customers, we have acquired tremendous experience in machinery applications. We want to combine this knowledge of application mechanics and control knowledge with AI based software expertise and solutions to lead the way in creating digital tools for our customers that enable them to exploit the latest technology advances.
The world is in a state of accelerating digital change. For companies like Festo within the automation technology sector, we must address these changes within our strategic approach to the future. Festo acquired the specialist software company Resolto to incorporate their latest machine learning, advanced analytics and artificial intelligence into our vision for the future. As a result, we developed Festo Automation Experience [Festo AX] to boost the performance of our customers' machines. It enables customers to make decisions based on the information and not just data through an easy-to-use software solution allowing users to extract value from the data produced by their equipment through artificial intelligence (AI) and machine learning.
A few years ago, there was a shift from the initial somewhat idealistic objective to monitor everything and anything on a machine. Experience shows it is better to home in on what is critical or has the greatest influence. It makes a lot more sense to identify priorities and focus on optimising the machine learning algorithms to spot data pattern anomalies within these areas first. It helps lift the fog within large data lakes and focuses on the areas with the fastest ROI. Pursuing a prioritisation strategy enables you to benefit from the quick wins and to work down the priority list with the payback examples already in-hand.
Analysing data & boosting productivity
We have gradually refined the key use cases. These are where users can best increase productivity, reduce energy costs, avoid quality losses, optimise their shop floor or create new business models by analysing and understanding their data with Festo AX. With pre-constructed modules for Predictive Maintenance, Predictive Energy and Predictive Quality, we have learnt that we can quickly and cost-effectively implement individualised solutions with our customers.
Festo AX does this by analysing data in real-time. It can be integrated flexibly into customers' systems – on premises, on edge or in the Cloud. The programs can run on Edge components directly by the machine, which has advantages in reduced latency. Costs for data transfer are reduced, meaning high-frequency data sampling and large data volumes are no problem. Customers frequently request this flexibility to ensure they achieve their cyber security requirements. No one other than themselves can access their data and solely access the collected analysis.
The data analysis is not limited to components and modules from Festo. A big advantage is that our Festo AX software makes it possible to analyse machinery incorporating components from many other manufacturers reliably; after all we don't expect the Festo components within a machine to be the ones that fail!
Diagnostics for maintenance
Predictive maintenance based on artificial intelligence offers additional advantages compared to traditional condition monitoring approaches. Increasingly data from the machinery can be merged with process data and evaluated with analysis models and cloud-based solutions. Festo AX uses artificial intelligence (AI) to detect deviations from the normal state of your production machinery at an early stage. The result is reduced unplanned downtime, lower energy costs and increased efficiency. We have found through many projects that an AI project's success is not only about the software but the ability to relate it and apply it to the application environment. Understanding and using the customers' terminology enables the outputs to be understood and acted upon.
We have also observed the benefits of setting up Festo AX to monitor machine output data in parallel to the standard control architecture. It reduces the risk of overloading or slowing the existing machine control by separating the two functions. This is particularly valued in existing, running installations where replacement of the control system would be risky and costly in programming and re-commissioning downtime.
The data is accessed through standard protocols such as OPC-UA and MQTT where possible. It is then processed by algorithms based on Artificial Intelligence and Machine Learning in real-time, outputting notifications when the data differs from the "healthy state" of the asset (anomaly detection). The report informs you of the sensors and can even provide a recommended course of action. Festo AX is 'trained' using the operators' knowledge to classify anomalies so that the software knows how to react next time the same anomaly pattern occurs. The algorithms improve through this iterative (human-in-the-loop) process, and unwanted notifications are eliminated. It is a 'reward' and 'punishment' process where the algorithm is positively reinforced for good reports and refined for those the operator doesn't judge as helpful. The more frequently the anomalies are generated, either by repetition across many identical machines or a high re-occurrence rate, the faster the digital model learns.
Once the optimisation cycle is underway, anomalies are detected very early, meaning that unplanned downtime is avoided, spare parts are ready, and maintenance is conducted without harming production.
Additional tools
We have also developed our offer from only connectivity and visualisation through dashboards a few years ago to Festo AX, which offers additional tools to help you discover new correlations in your data. For example, a root cause analysis can be automatically generated for every abnormal behaviour of your machine, and it shows which sensors are crucial for anomaly detection. The additional data visualisation enables you to get to the bottom of the anomalies and recognise critical correlations. You can additionally tune or optimise your application's algorithms within Festo AX by setting individual parameters.
We can supply our suggested default parameters but depending on individual needs, customers can easily adjust these in the user interface without in-depth or data science knowledge. You can document, manage, and forward anomaly detection outputs with the notification function. When an anomaly is detected, a notification is generated, including the following functionalities: data visualisation of the anomaly, automatic root cause analysis, and diagnostic and classification tool.
As mentioned earlier, depending upon the application, customers can choose between On-Premises, Cloud or Hybrid installations. With an On-Premises solution, the learning of the models takes place within your local control installation. For Cloud-based solutions, users can select their preferred environment or utilise space within the Festo Cloud space. A hybrid solution can also be established where complex computing tasks and large quantities of data are handled locally. Only defined outputs and the algorithm model training are outsourced to scalable cloud installations.
AI technology will continue to evolve extremely rapidly. This means that learning and experience need to be acquired as early as possible to gain maximum benefit. But it also doesn't make sense to justify large, all-encompassing block-buster installations. Instead, take an agile approach and identify the quick wins. The most successful projects have taken a staged approach – proposing and testing a hypothesis with a pilot evaluation and then upscaling from the learnings gained.
I look forward to seeing how this exciting technology evolves in the next few years.
- Proportional valve use whitepaper
- New standard in precision positioning
- Advanced dynamics
- Swimming robot inspired by nature
- Award winning collaboration
- INTEGRATING ELECTRIC AND PNEUMATIC CONTROLS
- Focus on smarter automation
- Automated biomass cultivation
- Pneumatic robotics meets artificial intelligence
- Productivity concepts
- EXTEND MOBILE INTO MAINTENANCE
- Complete XY Measuring Chain
- MONITORING FOR AUTOMATION
- IO-LInk Now Included
- As Long As The Wind Blows
- CONDITION MONITORING FOR THE COMMON MAN
- Central management of IP-based communication networks
- Breakdown Avoided Thanks To Condition Monitoring
- Magflow Meters
- Gas and Oil Automated